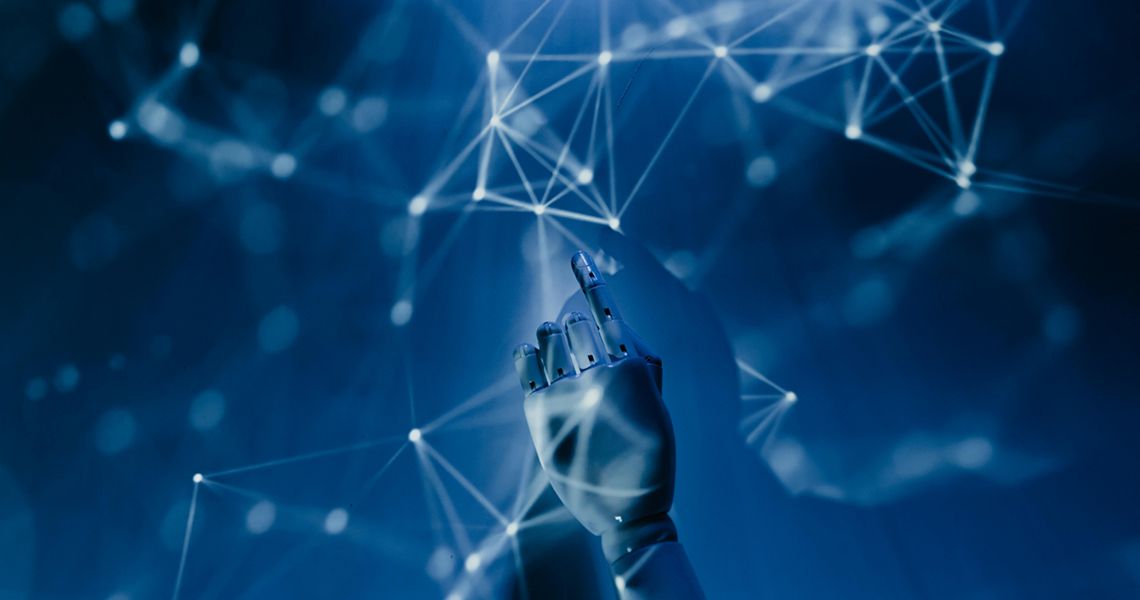
Artificial Intelligence (AI) has the potential to improve healthcare outcomes, yet AI technologies
can also pose risks to individuals, communities, and society. The burgeoning field of ethical data
science provides a venue for exploring the causes of bias in AI, and how to correct them,
rendering AI output as true, realistic, and fair to all demographic and socio-economic groups.
This workshop will focus on several major facets of mitigating AI risks, drawing from legal,
machine learning, statistical, mathematical, and clinical perspectives. It will include (a) a panel
discussion where experts will present challenges and latest advances on the topics of public trust,
transparency, statistical insight, and parity related to AI; (b) a poster session, including lightning
talks; and (c) subgroup discussions, where attendees may add their knowledge and ideas to the
dialogue on bias in AI, in use cases, legal and policy issues, and computational approaches to
improve data quality and prediction parity. We will conclude with a wrap-up of the workshop’s
findings and potential steps going forward, for both individual attendees, and for the overall
mitigation of bias in AI.
Visit AIME 2024 to learn more about the conference.
Format
The workshop will comprise two sessions divided by a 15-minute networking coffee break which include brief 1-minute poster presentations. At the end of the second session, a 15-minute discussion will be led by the workshop co-chairs to summarize the workshop findings, to have presenters to answer any additional questions, and to plan future directions.
Call for Submissions - Key dates
Submission open
April 4th, 2024
Submission deadline
May 30th, 2024
Notification of acceptance
June 5th, 2024
Mitigating AI Risk through Ethical Data Science Workshop
July, 2024
Review Process
The submissions will be reviewed on a rolling basis. Review results are expected to be communicated within 10 days after submission.
Topics
- AI risk
- Bias in AI and its mitigation
- Ethical data science
- Autonomy
- Trust
- Transparency and explainable AI
- Prediction parity and vulnerable populations
- AI and aging populations
- Chatbots
Submission Guidelines
Mitigating AI Risk through Ethical Data Science features poster submissions and should be submitted as a PDF file using our Easychair page according to the following guidelines:
Poster Abstract Submissions:
- 1 to 2 pages consisting of a structured extended abstract
- The structured abstract should include methods, results, discussion and references.
- Arial or Times New Roman font, size 11 are recommended.
- Figure and/or tables may be included as a part of the structured abstract.
Chairs
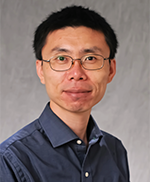
Yijun Shao, PhD
Dr. Shao earned his PhD in mathematics from the University of Arizona in 2010. He received Daniel Bartlett Fellowship in 2009 and Outstanding Research Fellowship in 2010 from the Department of Mathematics, University of Arizona. Dr. Shao completed a postdoctoral fellowship in biomedical information. His work has received won the Distinguished Paper Award in national and international conferences. His main research interest is to apply mathematical knowledge and skills to solving medical informatics problems. He has developed novel deep learning approaches and explainable AI methods. The explainable AI methods developed by Dr. Shao has been validated using clinical and synthetic data and compared favorably to other current methods. Dr. Shao presented, and led group discussions in an earlier presentation
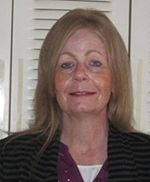
Terri Elizabeth Workman, PhD
Dr. Workman is an Associate Professor in the Department of Clinical Research and Leadership at The George Washington University. She earned a PhD in Biomedical Informatics from the University of Utah in 2011. Her current work has especially focused on underserved populations in healthcare, especially the LGBTQI community. Dr. Workman and her colleagues have developed novel methodologies to identify new risk factors in disease, identify patients at risk for suicide and self-harm, improve surveillance systems, understand temporal processes in disease pathologies, identify and extract symptoms, improve NLP tools, and increase insight into healthcare communications and processes. Dr. Workman also has extensive interest in teaching and service. She has been a co-instructor in several workshops in natural language processing, statistical modeling, and artificial intelligence. Dr. Workman coordinated the poster session and led group discussions in an earlier presentation of this workshop. She is supported by the George Washington University to attend this workshop.